Connections
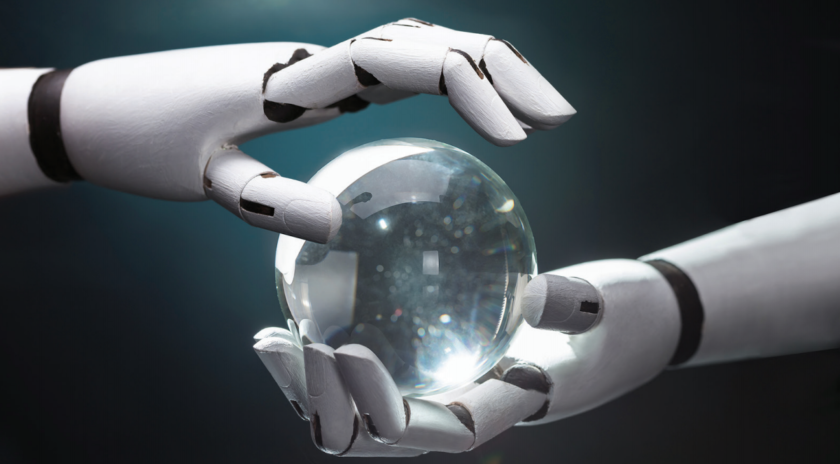
M&E Journal: Making Artificial Intelligence Real for Broadcast and Cable
Story Highlights
By Shiv Sehgal, Chief Product Officer, RSG Media –
Artificial Intelligence (AI) is all the rage. Everybody is talking about it and many companies are doing it: Google, IBM, Microsoft – all of the big names – and many startups to boot. And, while these companies are paving the way for AI, why does it feel like broadcast and cable companies are not actually using it? Can you think of an area where your company is really benefiting from AI?
First of all, it is important to understand that today’s AI technologies are not business applications. Rather, they are AI frameworks on which applications can be built. To use a metaphor of building a house, these frameworks provide the raw materials and the tools – the cement, lumber and the hammers. We need to leverage these tools to build the broadcast and cable house.
So, what does this house look like for companies in the sector? What is the real use case where AI can provide step-change improvement for the business?
Getting back to first principles
Above all, the ultimate goal of a broadcast or cable company is to grow its audience. If it can grow a large audience, it can command higher affiliate fees and earn higher ad revenue.
So, if the metaphorical house is a humming organization that is growing its audience and generating more revenue, then the foundation is an ability to accurately predict audiences.
If you can predict audiences well, then you will make better decisions in every process including content acquisition, program scheduling, marketing, and ad sales.
AI is effective at finding patterns in large datasets. The AI then uses those patterns to predict the future. This type of AI is called supervised learning and is a type of machine learning.
In the world of broadcast and cable, we are rich with historic data including ratings, program schedules, and heaps of viewership information. So, it is a perfect use case to leverage supervised learning.
How it works
It all starts with the inputs, the metadata. The algorithm considers all of the available metadata, including show names, premiere indicators, and competition. It then reviews years of the historical results: Nielsen ratings.
The magic of AI is in how it creates the relationship between the metadata and results. It trains itself to figure out how each piece of input data (technically known as a feature) impacts the ratings. In which direction does each piece of data move the ratings? To what significance? How do different data interrelate?
Once it figures out the relationship between inputs and outputs, it then has the model to predict the future. Feed the future input data into the model and it will predict the future ratings.
The algorithm trains itself to a complexity that goes far beyond analyses that we mere humans can comprehend, let alone construct. It often comes up with counterintuitive conclusions. As a result, it can produce far more accurate results, with much less manual work than traditional processes.
Leveraging AI in the business world is very challenging because of a gap in understanding. Young data scientists coming out of college don’t understand real business problems and business folks with years of experience don’t understand the new AI techniques or even the art-of-the-possible.
The biggest mistake that an organization can make is to hire a young data scientist, throw a problem at them and expect them to figure it all out without support. In fact, the algorithms are the easy part – it is tuning them to the business process that is challenging. It is incorporating all of the edge cases and nuances that makes the algorithm come to life (don’t worry – it isn’t really coming to life).
Another challenge is training business users to understand how to use the AI. We need to teach the business that the AI is just another tool in its toolbox. And, despite the “intelligence” moniker, the algorithm does no thinking – it is just math. The end user needs to understand where the algorithm has shortcomings and how it needs to be augmented with experience and institutional knowledge.
The secret to success in leveraging AI
So, if you want to leverage the technology, and make step-change improvement for your organization, don’t just hire a young whippersnapper data scientist and expect everything to fall in place. The secret to success is educating your business users on the art-of-the-possible of AI.
Give the business users a “101” overview of where AI algorithms shine, how they work in different industries, what type of problems they solve in the real business world. If you train your business users on the art-of-the-possible, they will come to you with the ideas. At that point, you can hire a data scientist or AI consultancy. Partner them up with the business user to co-lead the project.
If you follow this formula, you will solve the right problem, and have a game-changing tool that can be used in the real world.
Downstream impacts
To bring it back around to our real-world example for broadcast and cable, when you leverage AI in the right way, the ripple effect can be significant. In our use case, many downstream business processes will leverage our AI’s predictions:
* Content acquisition. You will be able to buy the right content because you can predict whether it will generate the right audience.
* Program scheduling. You will build optimized schedules because you can tune them to maximize your predictions.
* Ad sales. You will sell more effectively in the upfront and scatter markets because you will have accurate estimates of your impressions supply.
* Stewardship. You will deliver your deals more efficiently.
* Financial forecasting: You will be able to report accurate ad sales revenue forecasts to Wall Street because it is tightly coupled to your accurate audience predictions.
In conclusion, if you leverage AI to solve the right business problems, the rest of your business will follow. For broadcast and cable, it starts with ratings predictions. But, the AI doesn’t have to stop there.
This is just the beginning.
———–
Click here to translate this article
Click here to download the complete .PDF version of this article
Click here to download the entire Spring/Summer 2018 M&E Journal