Connections
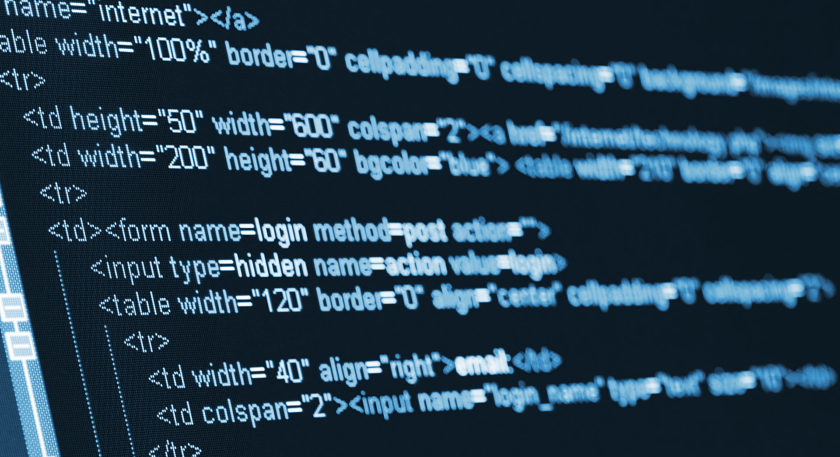
M&E Journal: How Entertainment Metadata is Adapting to Meet the Needs of Today’s Digital Viewers
Story Highlights
By Roz Ho, SVP and General Manager, Consumer and Metadata, TiVo –
With the rise of streaming services like Netflix and Amazon Prime Video, and the billions of dollars these services spend on original programming, there is more content available than ever. But viewers are struggling to wade through it all. In fact, it would take a single viewer more than 5 million years to watch the amount of video that crosses global IP networks each month, according to a recent Cisco report.
This tsunami of entertainment is making it harder for consumers to find content they actually want to watch based on their personal preferences, presenting a challenge for content owners and distributors: how to get return on the billions of dollars spent on digital content if it can’t be easily found, watched, and shared — today and in the future?
The value of metadata
That’s why it’s imperative to make it simple for viewers to search, discover and view content. But to do that, content owners need to know precisely what’s in their content. And to do that they need better content metadata, to improve the entertainment experience and to allow them to stand out in a crowded marketplace.
Content metadata is descriptive programming information, such as title, actors, description, release date, running time and genre. Enhanced metadata goes much further, with rich imagery, detailed actor background and an understanding of thematic elements or storyline, which is extremely valuable in improving content discovery for consumers.
The rapidly evolving digital media landscape compels content owners and distributors to understand and define their content metadata at a much deeper and granular level. In the movie The Big Short, for example, terms such as “hedge fund,” “real estate” or “mortgage” are thematically very relevant to the film, but not completely intuitive elements in traditional search systems.
Enhanced metadata that is automatically tagging these terms and other deep descriptors allows content owners to quickly create connections and serve customers faster and at scale. This deeper metadata is vital to providing accurate search results and intelligent recommendations, but mastering the art of metadata is proving to be challenging.
Large content owners, for example, usually have a long-tail catalog they need to monetize. These catalogs might not be up-to-date, lacking the right imagery or deep descriptors to support various search capabilities available today. Smaller content owners, for their part, are also under pressure to monetize their content. With tight budgets and tighter deadlines to deliver this content, they don’t have the time or expertise to enrich their metadata properly. Either way, the result of poor quality metadata can diminish customer experience and the content owners’ ROI.
The need for machine learning
TiVo’s approach to metadata helps address these problems by leveraging machine learning capabilities to help content owners and distributors increase the likelihood of content being discovered and ultimately viewed. By combining machine learning with expert human curators, we can create a set of weighted connections and keywords across vast content types to filter out “noise” and provide relevant and meaningful connections to video entertainment. TiVo is among the first to use machine learning techniques in its metadata process to surface the most relevant content, quickly and at scale, to create strong connections to content.
Machine learning takes basic metadata beyond cast, title and descriptions, and enables content to be enhanced with an extensive set of new data descriptors such as keywords, dynamic popularity ratings and moods. Machine learning can analyze large amounts of unstructured data and cut through the “noise” to identify and tag the relevant data to map to keywords and deep descriptors, automatically creating connections.
The power of voice
Machine learning can also enable conversational search and voice features, by leveraging the rich data that was automatically mined and deduced by machine learning. Viewers are starting to expect highly personalized, voice-enabled conversational interfaces that can anticipate intent and connect them with the right content using natural language.
Consider sporting events: A viewer could ask, “How are the Giants doing in baseball?” and the system can zero down to San Francisco Giants by using the connection between that team and baseball. This eliminates the NY Giants football team and provides a more accurate result.
Enriching content metadata to drive value
Now that consumers have more choices for content, one big question remains for creators: How do I get a return on my investment? How do I get maximum value from my traditional long-form content, my back catalog of content and even my new digital-first content?
The power of machine learning is that it can analyze large amounts of unstructured information and determine the relevant data to map to, thus making that content more discoverable, viewable, timely and valuable.
It can also enrich the content metadata, completing missing fields and tagging the content with deep descriptors to enhance discover-ability. By leveraging machine learning, metadata can adapt quickly to changes and continue to extract information, even when the structure changes like in the case of a redesigned website. Machine learning tools can automatically identify changes, adapt and learn for the next time, without slowing down.
Man and machine
Machine learning is clearly central to the future of enhanced metadata. Still, the need for human editors is not going away any time soon. As content genres get more specific—such as Netflix’s highly specific subgenres like “Steamy Crime Movies from the 1970s”—the need for human experts to curate this content also increases.
Think about how sharing-economy companies like Lyft or TaskRabbit can leverage technology to bring together the workforce they need at the exact location and exact time they need it, seamlessly mapping workers to relevant tasks. The same principle holds true for editors of metadata. If you want your catalog of “Emotional Independent Dramas for Hopeless Romantics” to thrive, you’d prefer true aficionados of the genre editing that content, because they can do a much better job than anyone — or anything — else.
As the volume and breadth of content continues to explode, machine learning-powered metadata is a good scaled solution to create new and unprecedented revenue opportunities. It can do this by highlighting untapped entertainment for content creators and distributors, and driving simpler and more personalized entertainment experiences for viewers. So, sit back and enjoy the show!
—-
Click here to translate this article
Click here to download the complete .PDF version of this article
Click here to download the entire Spring/Summer 2018 M&E Journal